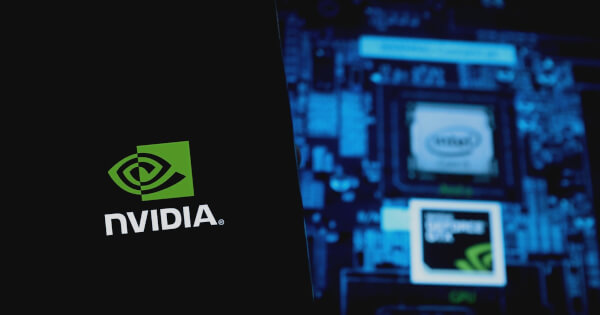
NVIDIA has unveiled Hydra-MDP, an modern framework designed to advance the sector of end-to-end autonomous driving. In accordance with the NVIDIA Technical Weblog, Hydra-MDP leverages a multi-teacher, student-teacher data distillation structure to reinforce the scalability and security of autonomous driving techniques.
Groundbreaking Framework for Autonomous Driving
Constructing an autonomous system that effectively navigates advanced environments whereas making certain passenger security and luxury is a big problem. Hydra-MDP addresses this by integrating data from each human and rule-based planners, permitting the mannequin to be taught various trajectories and enhance generalization throughout numerous driving situations.
This common framework showcases how machine learning-based planning may be bolstered by rule-based planners, making certain that the mannequin not solely mimics human driving behaviors but in addition adheres to site visitors guidelines and security requirements. This integration addresses the constraints of conventional imitation studying methods.
Knowledge-Pushed Scalability and Robustness
Hydra-MDP’s data-driven scaling legal guidelines underscore its robustness and flexibility. By using pretrained basis fashions with in depth datasets and GPU hours, Hydra-MDP demonstrates its potential for steady enchancment. The framework’s scalability was highlighted when it gained first place and the innovation award on the E2E Driving at Scale Problem at CVPR 2024, outperforming state-of-the-art planners on the nuPlan benchmark.
Key Classes and Improvements
Hydra-MDP’s improvement has offered a number of crucial insights that formed its structure and success:
- Embrace Multimodal and Multi-Goal Planning: Conventional techniques typically give attention to single-modal and single-target goals, limiting their real-world effectiveness. Hydra-MDP integrates various trajectories tailor-made to a number of metrics, together with security, effectivity, and luxury.
- Make the most of Multi-Goal Hydra-Distillation: By using a number of specialised academics (each human and rule-based), the mannequin predicts trajectories that align with numerous simulation-based metrics, enhancing generalization throughout various driving situations.
- Overcome Publish-Processing Limitations: Hydra-MDP integrates notion and planning right into a seamless pipeline, sustaining the richness of environmental information all through the decision-making course of.
- Incorporate Environmental Context: Utilizing options from LiDAR and digital camera inputs, Hydra-MDP’s notion community higher understands and reacts to advanced driving environments.
- Iterative Refinement via Simulation: In depth offline simulations generate floor reality simulation scores, which supervise the coaching course of, bridging the hole between theoretical efficiency and real-world applicability.
- Efficient Mannequin Ensembling: Methods like Combination of Encoders and Sub-score Ensembling mix mannequin strengths, enhancing robustness and dealing with a various array of driving situations with excessive accuracy.
Conclusion
The event of Hydra-MDP represents a big leap ahead in autonomous driving know-how. By embracing multimodal and multi-target planning, leveraging multi-target hydra-distillation, and refining via in depth simulations, NVIDIA has created a mannequin that considerably outperforms current state-of-the-art strategies. These developments provide a promising roadmap for the way forward for AI-driven autonomous techniques.
For extra info, see the total particulars on the NVIDIA Technical Weblog.
Picture supply: Shutterstock