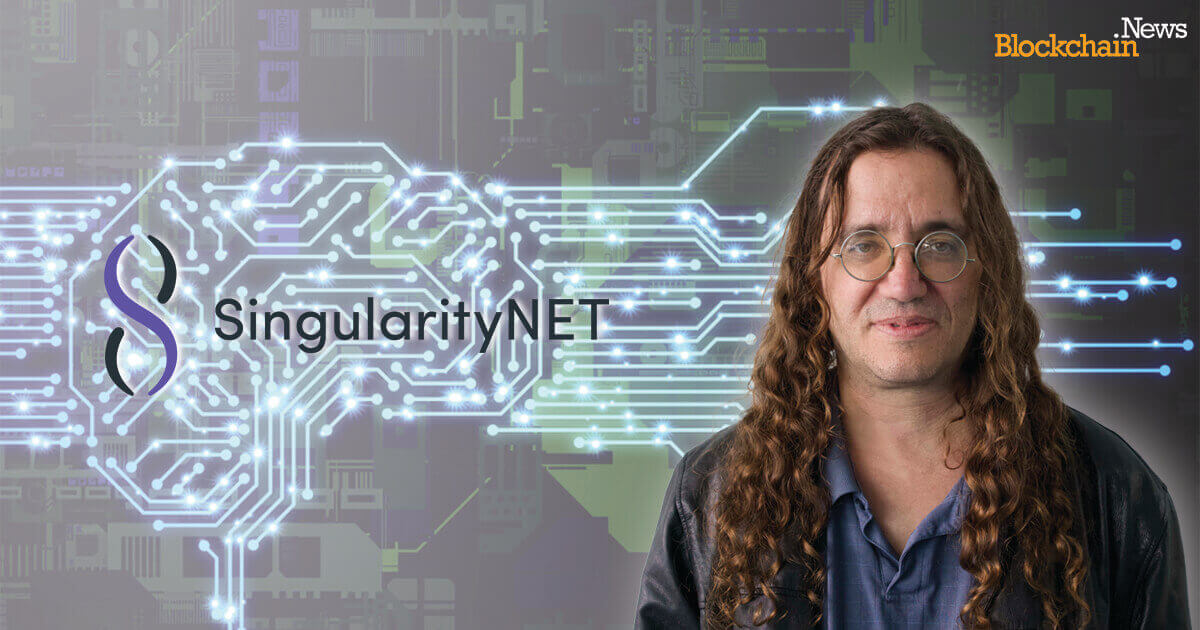
Regardless of vital developments, present deep studying fashions stay essentially restricted of their capability to attain synthetic basic intelligence (AGI), in line with a latest evaluation by SingularityNET (AGIX). Whereas these fashions have revolutionized synthetic intelligence (AI) by producing coherent textual content, sensible photos, and correct predictions, they fall brief in a number of essential areas needed for AGI.
The Limitations of Deep Studying in Attaining AGI
Lack of ability to Generalize
A serious criticism of deep studying is its lack of ability to generalize successfully. This limitation is especially evident in edge instances the place fashions encounter eventualities not lined of their coaching knowledge. As an example, the autonomous automobile business has invested over $100 billion in deep studying, solely to see these fashions battle with novel conditions. The June 2022 crash of a Cruise Robotaxi, which encountered an unfamiliar situation, underscores this limitation.
Slim Focus & Information Dependency
Most deep studying fashions are designed to carry out particular duties, excelling in slim domains the place they are often educated on massive datasets related to a specific downside, resembling picture recognition or language translation. In distinction, AGI requires the flexibility to know, be taught, and apply information throughout a variety of duties and domains, much like human intelligence. Moreover, these fashions require monumental quantities of knowledge to be taught successfully and battle with duties the place labeled knowledge is scarce or the place they must generalize from restricted examples.
Sample Recognition with out Understanding
Deep studying fashions excel at recognizing patterns inside massive datasets and producing outputs primarily based on these patterns. Nonetheless, they don’t possess real understanding or reasoning talents. For instance, whereas fashions like GPT-4 can generate essays on quantum mechanics, they don’t perceive the underlying ideas. This hole between sample recognition and true understanding is a major barrier to attaining AGI, which requires fashions to know and motive about content material in a human-like method.
Lack of Autonomy & Static Studying
Human intelligence is characterised by the flexibility to set objectives, make plans, and take initiative. Present AI fashions lack these capabilities, working inside the confines of their programming. In contrast to people, who repeatedly be taught and adapt, AI fashions are usually static as soon as educated. This lack of steady, autonomous studying is a serious hindrance to attaining AGI.
The “What If” Conundrum
People interact with the world by perceiving it in real-time, counting on current representations and modifying them as needed for efficient decision-making. In distinction, deep studying fashions should create exhaustive guidelines for real-world occurrences, which is impractical and inefficient. Attaining AGI requires transferring from predictive deductions to enhancing an inductive “what if” capability.
Whereas deep studying has achieved exceptional developments in AI, it falls wanting the necessities for AGI. The constraints in understanding, reasoning, steady studying, and autonomy spotlight the necessity for brand spanking new paradigms in AI analysis. Exploring different approaches, resembling hybrid neural-symbolic programs, large-scale mind simulations, and synthetic chemistry simulations, might deliver us nearer to attaining true AGI.
About SingularityNET
SingularityNET was based by Dr. Ben Goertzel with the mission of making a decentralized, democratic, inclusive, and helpful Synthetic Basic Intelligence (AGI). The SingularityNET workforce consists of seasoned engineers, scientists, researchers, entrepreneurs, and entrepreneurs, with specialised groups devoted to numerous utility areas resembling finance, robotics, biomedical AI, media, arts, and leisure.
For extra info, go to SingularityNET.
Picture supply: Shutterstock